Sometimes Papers Contain Obvious Lies
What you read in an abstract, a title, or even in the body of a paper might be the opposite of what papers actually show, and sometimes result are just made up
This post is brought to you by my sponsor, Warp.
This was a timed post. The way these work is that if it takes me more than one hour to complete the post, an applet that I made deletes everything I’ve written so far and I abandon the post. You can find my previous timed post here. I placed the advertisement before I started my writing timer.
The authors of scientific papers often say one thing and find another; they concoct a story around a set of findings that they might not have even made, or which they might have actually even contradicted. This happens surprisingly often, and it’s a very serious issue for a few reasons. For example:
Most people don’t read papers at all, so what they might hear is polluted second-hand information, and if they do read papers, they usually just read titles and abstracts, and those are frequently wrong.
People trust scientists and scientific institutions, and they should be able to trust scientists and scientific institutions. If that trust is broken, an important institution can lose a lot of its power for doing good.
People trust peer reviewed works, and they should able to trust in the power of peer reviewed works. If that trust is broken (and it should currently be), a potentially important tool loses a lot of its power for doing good.
Scientific institutions trust scientists, and they allocate funding based on the things scientists tell them. assuming they’re trustworthy, so when that trust is violated, they’re liable to misallocate funding and research effort.
And the list goes on…
At times, this violation of trust is so egregious that it’s easy to wonder how it could have become publicly known in the first place. Ideally, outright lying is something that gets caught in peer review, or which people are just too ashamed to do. But though you might think better of scientists than so many of them have proven to be, plenty of them do still brazenly lie.
Lying in scientific papers happens all the time.
For example, last year I dissected a paper that several newspapers had reported on which alleged that physically high ceilings in test-taking locations made students score worse on their exams. This paper’s abstract, title, text, and the public remarks to journalists from the authors all implied that was what they found, but their actual result—correctly shown in a table in the paper and reproducible from their code and data—was the complete opposite: higher ceilings were associated with higher test scores! Making a viral hubbub about this managed to get the paper retracted—eventually—but the retraction notice barely mentioned any of the paper’s problems and, instead, said that whatever issues warranted retraction were examples of good ole “honest error”.
Usually when a paper contains lies, they’re hidden a bit better than that. Consider the concept of an Everest Regression. We know Mount Everest is very cold, but a clever person can argue that it’s not cold, once you control for its high altitude. The high altitude of Mount Everest is why it’s cold, but that doesn’t matter. To the researcher claiming Mount Everest is actually warm, all that matters is the result of their ‘analysis’, where they’ve ‘controlled’ for a variable that is, incidentally, the key explanatory variable in this case. This can also be extended so, for example, McDonald’s is equivalent to a Michelin 3-Star restaurant after controlling for the quality of ingredients, preparation, ambience, and price.
If the responsible researcher is ignorant of this issue, they’re not lying, they’re just mistaken. But if they’re aware of what the problem is and they are nonetheless insistent that Mount Everest is actually warm without qualification, then they’re lying. As it happens, plenty of researchers lie in just such a way. Any economist worth their salt will not find themselves conducting an Everest Regression, and yet, when it’s convenient, they’ll use them if they want to persuade people.
A pair of researchers published a paper on immigration and crime in Germany days before the recent election. Their conclusion? More foreigners does not increase the crime rate.
Immigrants in Germany have a vastly elevated crime rate compared to natives, and this is true across the board, from theft and fraud to rapes and sexual violence to homicide and other violent crimes. Consistent with this, the paper reports that the higher the proportion of foreigners in a district or region (pictured below), the higher its crime rate:
But, after controlling for differences in age, sex, suspect rate, unemployment, and taking out some fixed effects, immigration at the district or region level is no longer related to crime. But wait! Why did they control for the suspect rate? That is, in effect, controlling for the dependent variable to then say that foreign-born population shares are unrelated to crime. But if they drive differences in crime across different parts of Germany, this is just controlling for altitude and declaring Everest is hot, stating that stadiums make people run fast!
Ignoring, for a moment, that the authors used a very inappropriate control that trivializes their results, they were not equipped to produce a causally-informative result in the first place. What their paper entails doing is, instead, proposing a strong ecological hypothesis, where differences between regions drive differences in criminality, and foreigners happen to be situated in places with high crime due to some sort of sorting mechanism. They then sought to show that there’s some data that can fit with this hypothesis. What they did is, thus, arguably, appropriate, but to believe that, you have to believe the model, and the evidence from outside of the exercise they presented fits exceptionally poorly with their proposed model.
In order of increasing relevance and debunky-ness, consider these results:
Using individual-level data, immigrants in Sweden remain more likely to commit rapes even after controlling for much better measures of socioeconomic status, like individual welfare receipt, income, and neighborhood-level deprivation, and even for prior criminal behavior and all of alcohol use disorder, drug use disorder, and having any psychiatric disorder. Because this is individual-level rather than district or region level data, it should be considered more convincing than the ecological exercise from the German crime paper, but if one believes a strong ecological model, it may not be.1
Ecological models of the determination of crime are not supported by the large, causally informative studies that have been done on them. Ecological models have not held up well at all, and what remains of ecological hypotheses is, at best, very weak effects which are nowhere near sufficient to explain immigrant overrepresentation in crime in Germany.
Causally-informative evidence from Germany that exploits administrative refugee assignments to estimate immigrants’ impacts on crime shows that they do, in fact, increase crime.2 But the crime-producing effect of refugee settlement is lagged, meaning it doesn’t happen instantaneously. This effectively adds insult to injury, because by its nature, a lagged effect adds evidence that the cross-sectional ecological model is inappropriate.
The authors of this paper produced a compromised analysis that doesn’t hold up and they made it painfully obvious that their findings were politically motivated. Quoting them:
This finding fuels the concern that migration could endanger security due to a higher tendency for criminality among foreigners. Security concerns are a central argument in the current election campaign for limiting immigration. For example, Bavarian Prime Minister Markus Söder stressed at the CSU winter retreat that migration must be “limited and thus internal security improved”. The CDU/CSU’s candidate for chancellor, Friedrich Merz, believes that this is “bringing problems into the country” and calls for the revocation of German citizenship for criminal citizens who have a second nationality.
This paper was written less as part of a truth-seeking effort, and more to accomplish a political goal, to provide evidence that one of the biggest issues to voters in a forthcoming election wasn’t really much of an issue at all. This objective and the obviousness of the issue to anyone with a modicum of competence makes this paper a pretty clear example of scientists lying and engaging in an attempt at gaslighting.
But there’s worse out there. As I’ll show, scientists often lie with far more brazenness.
A Message From My Sponsor
Steve Jobs is quoted as saying, “Design is not just what it looks like and feels like. Design is how it works.” Few B2B SaaS companies take this as seriously as Warp, the payroll and compliance platform used by based founders and startups.
Warp has cut out all the features you don’t need (looking at you, Gusto’s “e-Welcome” card for new employees) and has automated the ones you do: federal, state, and local tax compliance, global contractor payments, and 5-minute onboarding.
In other words, Warp saves you enough time that you’ll stop having the intrusive thoughts suggesting you might actually need to make your first Human Resources hire.
Get started now at joinwarp.com/crem and get a $1,000 Amazon gift card when you run payroll for the first time.
Get Started Now: www.joinwarp.com/crem
Abstracts Are Frequently Poorly Related To Their Papers
In 1999, Pitkin, Branagan and Burmeister analyzed random samples of articles and their abstracts published in Annals of Internal Medicine, BMJ, JAMA, Lancet, NEJM, and CMAJ, several top-tier medical journals. They found that large portions of the papers published in those journals had reporting inconsistencies between what was in the abstract and what was in article bodies.
In Pitkin, Branagan and Burmeister’s study, “inconsistency” refers to an abstract and a paper’s body presenting statistics that do not agree with one another for reasons not due to chance, like rounding. For example, if my abstract says “We found 1+1=2” and my article says “We did not find that 1+1=2”, that’s inconsistent. They also looked at whether abstracts included omissions, which were defined as times when statistics were presented in abstracts and then never shown in the bodies of papers.
For one journal, the majority of papers (68%) had one or both or these errors; for all journals, the number with such issues was always sizable:3
Two of the authors of this research came back later and evaluated a randomized controlled trial that was intended to assess if the field could solve the problem by identifying specific errors authors made, during the review process. Despite the identification, few authors corrected their mistakes, and the intervention group saw 28% of abstracts being inconsistent versus 26% in the control group. After this, the full original team of researchers assessed the effectiveness of a policy adopted by JAMA, wherein the journal provided the impetus to be consistent to submitting authors. This policy seemed to be effective, as, pre-intervention, 52% of abstracts had inconsistencies versus 20% after the policy went into effect. Perhaps this was more effective than their earlier experiment because the journal staff were the ones providing notice about inconsistencies.
The finding that abstracts are reliably unreliable has been replicated umpteen times, across many fields, and there’s no doubt that a substantial number of the abstracts from studies published in practically any field will be inconsistent with their papers. We know this holds in fields as diverse as clinical chemistry, surgery [1], candidate gene studies, dentistry, psychiatry and psychology, pharmacy, and even biomedical research writ large [1, 2].
It’s possible to argue that these results indicate errors rather than evidence—prima facie or direct—of malfeasance. But many of these abstracts don’t just have benign errors, they contain spin4, which is hard to be anything but intentional. Other types of evidence for malfeasance are similar to this, in that they could be down to error, in part or in whole. Regardless of the cause, they’re still evidence of a need to place less trust in papers, and particularly in their abstracts. While these findings probably reflect a mix of both error and fraud, no matter: there’s plenty of direct evidence of fraud.
There Are Many (Frequently Obvious) Fraudsters
Thanks to alert sleuths and automated detection tools, some of the most obvious examples of scientific fraud are now being noticed at scale. A preprint from last year showed this for image duplication in preclinical depression studies.5 The three types of duplication the article looked into were simple duplications, duplications with image repositioning, and duplications with edits; visually, those look like this:
Type I image manipulation is the most likely to be innocuous, but it is still sometimes suspect. For example, sometimes images are repeated between papers and authors claim they’re from different experiments. This often happens in the cases of paper mills, where the people producing the purchased papers are lazily reusing assets for different customers.
Type II image manipulation can also be innocuous, but that’s less often the case. These involve taking an image and duplicating part of it while changing its orientation, zooming in, or making other mildly obscuring presentation choices. Unfortunately, these sorts of manipulations are usually not innocuous, even though there’s still plenty of opportunity for them to be. When they are innocuous, they often come with an explicit note, like “Image B is a zoomed-in version of image A.”
Type III image manipulation can rarely be innocuous. This manipulation involves duplicating an image but outright altering some aspect of it. This is usually done to make a fake finding, and rarely done to, say, increase the contrast to reveal difficult to see elements of images compared to their raw form.
Out of 1,035 papers screened for a systematic review of preclinical depression work, the authors of this study found that 588 included images, and 19% of those that had images featured duplicates. That's 19% with a very clear issue. Now maybe some of those are innocuous accidental duplications of the Type I variety. But if you look at the breakdown, it actually seems that there are more Type II and III, severe duplication events. That's bad, and this is a lower bound on how bad things are.
Before going on, do note: 17 “Other” problems, not fitting into the typical taxonomy. These were described as issues like images being switched around, leading to mismatched descriptions, and cases where images appeared to come from a "paper mill" that was fabricating research at the request of paper authors.
This is all bad, but what does it do? It’s somewhat hard to say. The studies that had duplication issues tended to have smaller variances and they reported 0.81 g larger effects than studies that weren't flagged, but though that’s biasing for a meta-analysis or review, they still fit in with plenty of the studies that didn’t have duplication issues:
Even when these problematic papers were stratified by their checklist-based “quality of reporting”, they seemed to be indistinguishable from the papers that weren't obviously fraudulent. Their “quality of reporting” came out at 5.8 out of 10 versus 5.1 out of 10 for all of the studies with a quality rating. The “risk of bias” wasn't much better, showing a score of 3.2 out of 11 for the potentially fraudulent studies and 2.9 out of 11 across every study where the risk of bias was reported.
This is an amazingly unfortunate finding. It indicates common metrics for indicating study quality don't pick up outright, visually-detectable problems. Or, maybe they do detect problems, and there are just huge numbers of undetected problems among remaining studies. That is the best case scenario, but it's not a happy one either! But surely peer reviewers, editors, and readers of studies will identify and penalize papers with image duplication issues, right? This is an analysis of published works, so the answer must be no to the question about peer review. But what about prestige? As it turns out, papers with image duplication issues are published in journals just as high-impact as papers without image duplication issues, and the number of citations to problematic papers is equivalent to the number of citations to ones that are not visibly problematic:6
The authors wrote:
We would suspect that the reason why most researchers would agree with the statement that research fraud is rare, all the while data seems to point to the contrary, is that they have not encountered it personally.
Perhaps there’s something to this. When researchers are explicitly asked whether they have doubts about the integrity of different groups’ research, they tend to state two things:
They don’t doubt their research or the research of their collaborators.
They often doubt the research of everyone else.
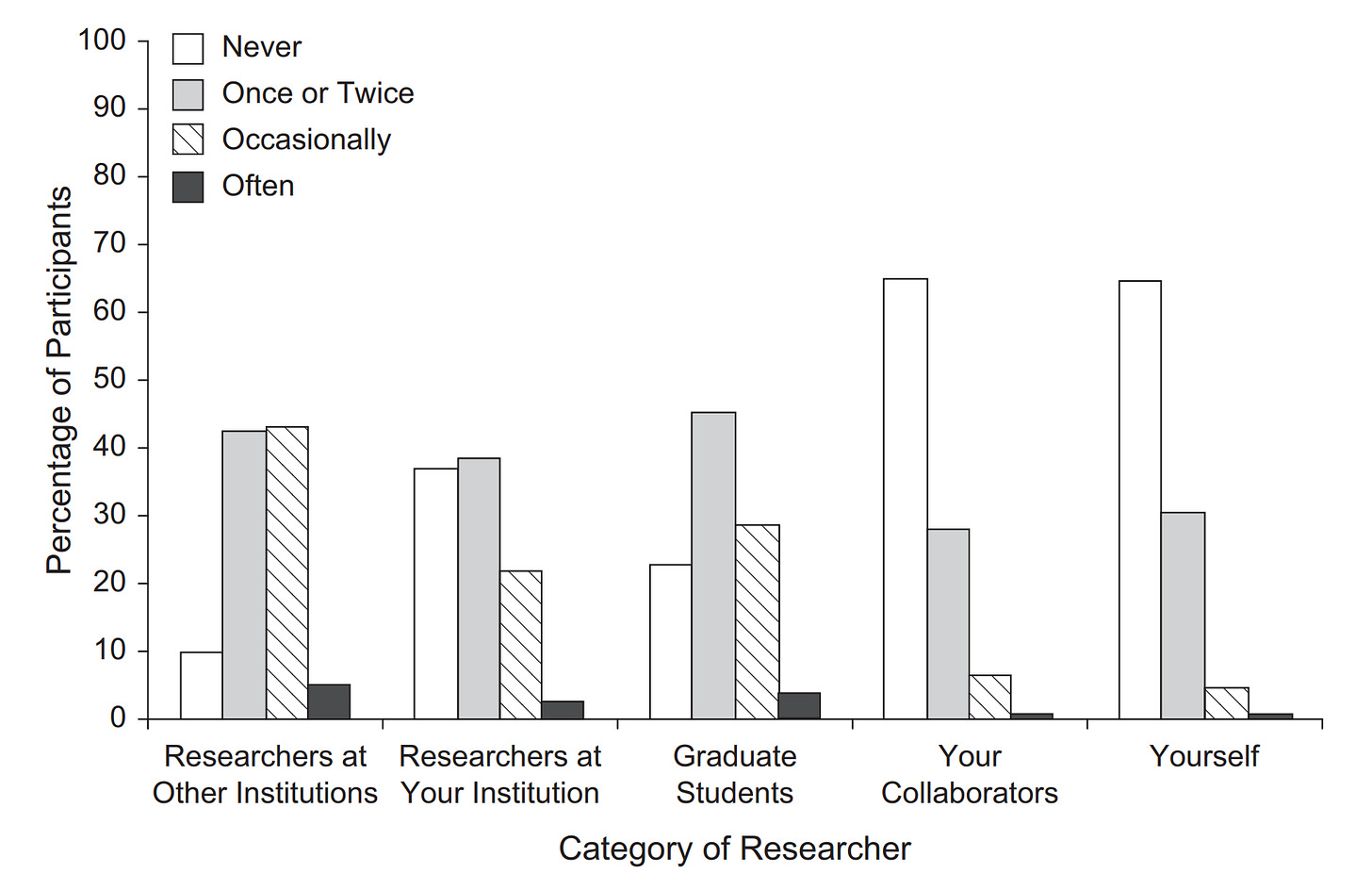
But hold on. Though this is what researchers say, if you ask them how often they engage in questionable research practices (QRPs), there’s widespread admission to problematic scientific behaviors:7
The prevalence of QRPs is too high to be consistent with people’s stated confidence in the integrity of their work, unless they just don’t care about QRPs like the ones listed here. This holds even looking at major QRPs like selectively reporting results. Regardless, the results of this survey are independent, major evidence that, if anything, the 19% image duplication rate I mentioned vastly undersells rates of misbehavior-driven problems in science, and this also adds considerable direct evidence that fraud is common.8
Needed: More Data, More Retractions, Better Mechanisms
A while back, I wrote about the case of an AER paper on dividend taxation that included a line in its code that showed the main result was fraudulent. The authors simply divided one of their coefficients to promote the appearance of a desired result. To make matters worse, their results were not robust and were driven by an unreasonable specification choice. The reason this fraud was caught is that papers in the AER are supposed to have replication packages—code and, hopefully, data to make their results reproducible. Looking through the code made the fraud apparent; having the data to reproduce the result proved the paper’s policy-relevant conclusions were not robust in any case.9
This sort of data sleuthing is only rarely possible for a simple reason: few papers have available data. To make things worse, few papers include the code required to reproduce their analyses, the ones that do tend to have really bad code,10 and it’s exceptionally rare to get both data and code at the same time. In the majority of cases across many fields, papers making the statement that ‘data is available upon request’ don’t even provide their data when it’s requested!11
The situation this leaves science in is not a favorable one. If we want to reliably fight fraud, data and code needs to be provided. There are plenty of techniques to find errors12 which might be indicative of fraud or at least low-quality research, but they might not lead to decisive enough indictments of bad research for actions to happen, and actions do need to happen, or people will be misled. This applies not only to the public, but to researchers who build on fraudulent work. For example, it took more than a decade for the authors of this meta-analysis to note that their results were polluted by the inclusion of fraudulent datapoints, through only slight fault of their own.13 There have been tons of (oftentimes very expensive) studies that attempted to replicate the results of fraudsters like Francesca Gino and Dan Ariely, and in the biomedical sciences, fraud has even provided the basis for clinical trials which, seemingly inevitably, fail.
To stop this, in addition to improved detection, we need improved retraction: more papers need to be pulled from the literature. The good news is that there are increasingly many retractions in even pretty far out fields.
The reasons for the increase have been linked to institutional changes that increased the ease of publication and retraction, but that study is now dated. I suspect the increase in retractions in more recent years reflects increasingly positive attitudes towards retraction. It remains the case that many researchers think that retractions are a punishment, but that view feels like it’s fading—and it should fade! It is an incorrect view, and retractions need to be used to clean up even majorly erroneous science, rather than just the transparently fraudulent variety.
The bad news is that retractions do not have the huge effects they should. Using data on over 1,100 scientific retractions, researchers found that the resulting impact on citations was small: a 5-10% reduction in the citation rate, with somewhat larger effects for studies retracted for fraud or misconduct as opposed to honest error. That’s something, but it’s not much.14 Retractions are nice, there should be a lot more of them, but something else must be done unless the effect of retractions dramatically changes.
I was recently introduced to the practical implementation of a partial solution in a paper I had the luck to review: journal-level mechanism design. A journal implemented automatic warnings to researchers if they cited retracted papers. The result? A significant reduction in citations going to retracted papers. What’s more, the citations to retracted papers became more negative towards them! This was not enough to fully address the issue of citing retracted works, but it’s a starting point, and I think it can inspire a much more complete solution set.
That solution? Designing and implementing more (hopefully cheap and easily deployed) mechanisms just like this one. Maybe abstract inconsistency can be identified with AI. Perhaps journals can all deploy tools to automatically detect and classify image duplication, and maybe someone can create a database of images from scientific papers to query more broadly. Papers that fail to provide their code could be automatically rejected because they’re suspect. Journals could host data instead of leaving it up to authors to choose when and to whom to provide it. There are tons of possibilities!
But until we start seeing anti-fraud measures becoming commonplace and science rapidly cleaning itself up of the fraud it’s been beset by, we’ll just have to live with and adapt to the fact that you can’t trust what papers say and researchers frequently lie.
Fundamentally, when an individual-level analysis ‘controls away’ an effect without some means of causal identification, it runs into the issue that the immigrants in reality still differ from post-control immigrants. ‘Explaining’ the difference between immigrants and natives adds basically nothing to any discussion unless the proposed explanatory variables are actually causally impactful and open to being altered.
One could argue that this paper is too theoretically distinct, because it specifically references the impacts of refugees, but those are most of the immigrants that people consider problematic, so the point is moot.
Unfortunately, we are not provided with the names to identify which journals are most problematic.
Which can matter.
Image duplication is the type of fraud that disgraced neuropathologist Eliezer Masliah was caught doing.
Because these studies are so hard to distinguish from ones that are not clearly problematic, it’s unclear if the studies discussed here contribute to the replication crisis. They might alongside additional fraudulent studies that didn’t have obvious image duplication issues, but that’s not established. Incompetence is likely a more common explanation for replication issues. For example, consider studies on the effects of experiencing poverty on cognition.
This study also provides prevalence estimates for Germany, but they were computed with an invalid method. The reason the method is invalid is that it understates the QRP rate. In the case of the Indonesian data, that method was applied to the data to see what the result would be, and the rate people admit they do fraud at was higher than the rate that was estimated. This is a clear indictment of the method, which is not to say that the other method is impervious to issues, merely that it isn’t prima facie unreasonable.
This is another image-based fraud detection study that I found highly interesting. Other studies dealing with the same categories of image duplication have produced interesting findings like that duplication rates vary by country. Retraction rates also vary by country, and they do not seem to vary with quality of scientific institutions but, instead, its reverse. This makes sense, because those places are likely to produce more retraction-worthy science.
The AEA looks good in this instance, but their data availability practices still leave a lot to be desired.
Academics are notoriously bad at programming.
It was still their fault for not being careful enough since the fraud was known at the time they did their meta-analysis, but this is a simple mistake and an abnormal occurrence. They’re hardly at serious fault, because it is generally not considered necessary to look into whether researchers who produced datapoints for meta-analysis were frauds.
The best solution is likely to be a detailed retraction in order to reduce misapprehensions based on the initial results, but another possibility is simply correcting the paper. The problem with just correcting the paper is that the results as they are alongside numerous copies of the faulty paper have already gone so far that the public and the research community are liable to keep being exposed to the original more than the follow-on if severe action isn’t taken.
It’s worth noting at this point that retraction notices are often not sufficiently detailed, and they frequently mislead about the quality of papers. For example, I mentioned this paper I dissected was retracted, but notice that the retraction notice barely touches on any of the material errors of fact in the paper and, instead, mentions honest errors. At a minimum, journals should publish a log of what happened during investigations into papers that got retracted.
The rewards for replication and the penalties for failed replication are also not that large.
Sometimes I wonder if I'm being lied to when the author assures me that his very thoughtful and well-structured post was written in under an hour.
In my experience, even well educated researchers have great difficulty understanding how QRPs can undermine the meaning of their results. In part, it is a matter of being "hard to get a man to understand something when his livelyhood depends on not understanding it." But also it is the difficulty in imagining that just a few QRPs can create an irreproducible, meaningless result. Perhaps all researchers should be required to perform sensitivity analyses even in fields where.that isn't the norm. I remember being very excited about a surprising result from a pilot project and getting ready to try to replicate it when I saw that the effect was entirely driven by a single extreme outlier.